Forecasting future may one day become as practical as predicting weather, thanks to Big Data advances
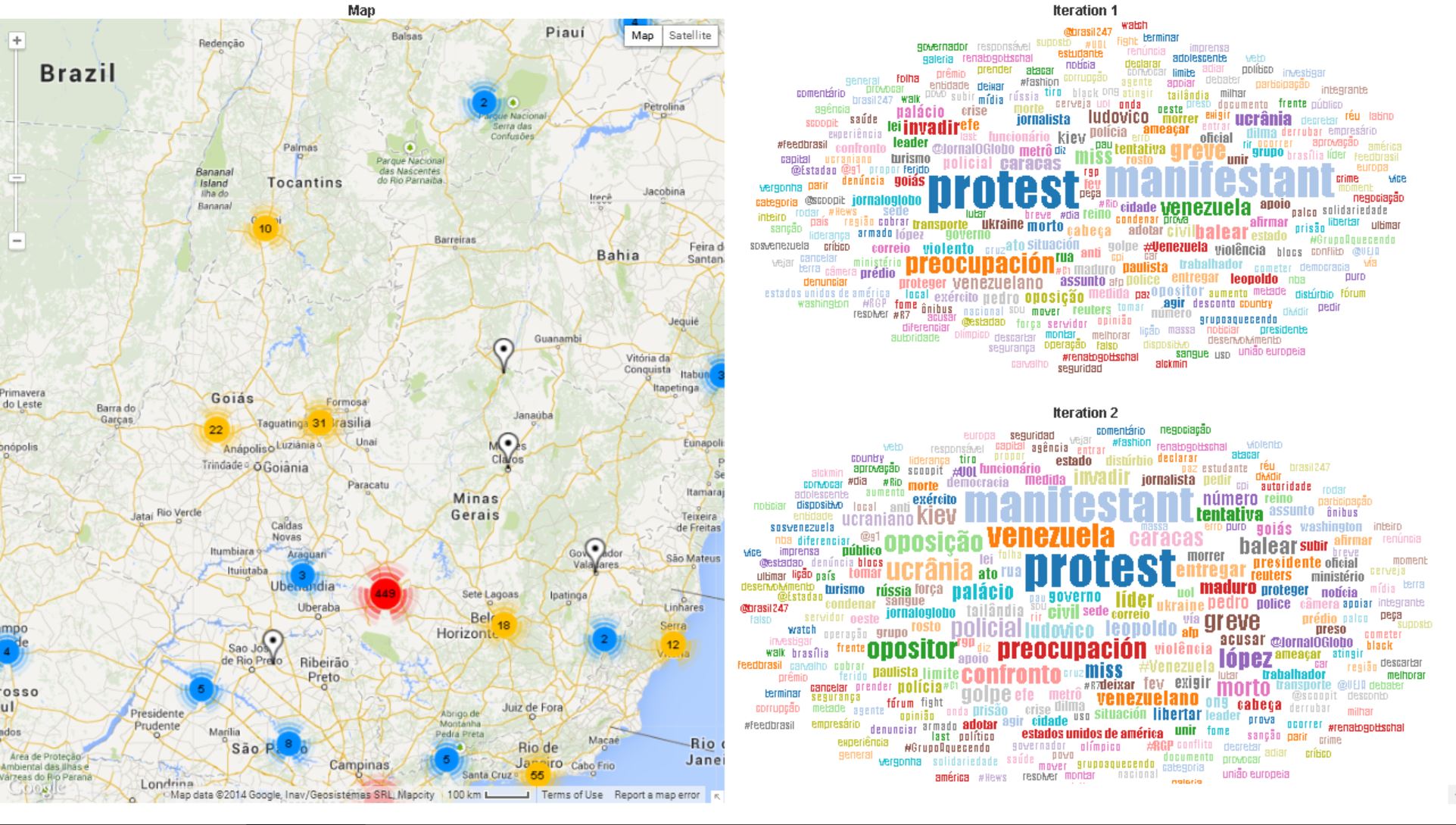
It was standing room only in a crowd of computer engineers, artificial intelligence experts, philosophers, and virtual reality gurus — all gathered around to hear how forecasting the future may one day be as commonplace as forecasting the weather, thanks to advances in the field of Big Data.
“There is essentially more data being uploaded than what we’re actually experiencing,” says panelist Devi Parikh, assistant professor of electrical and computer engineering, who leads the Computer Vision lab at Virginia Tech. “Every second of life produces somewhere near two hours of content.”
Parikh may have startled people unacquainted with high-volume data management and stream computing, but this day in Kelly Hall — home of the Institute of Critical Technology and Applied Science at Virginia Tech — Parikh was surrounded by believers.
After all, this is the home of the Early Model Based Event Recognition using Surrogates project, or EMBERS — Virginia Tech’s effort to harness this torrent of data to generate early warnings of events with a broad social impact.
And as Wu Feng, a professor of computer science and electrical and computer engineering, points out, “It is not just the volume of data we are interested in, but the veracity and variety as well.”
In the past year, the team has used such an approach to correctly forecast many important events such as the riots after Paraguay’s president was impeached, the Hantavirus outbreaks in Argentina, and the recent mass protests in Brazil and Venezuela.
As leaders of the only project to receive the final and third year of support from the Intelligence Advanced Research Projects Activity, the team was eager to share information about its achievements and plans for growth.
Project leader and director of the Discovery Analytics Center Arlington site Naren Ramakrishnan explained how all of the event alerts are emailed in real time and compared against a gold standard report organized by a third party. The center is supported by the Institute of Critical Technology and Applied Science and is based in the Department of Computer Science of the College of Engineering.
The team actually receives a report card scoring their predictions. They also test what they could have forecasted and determine what they might have overlooked.
Local project collaborators spawn from the departments of computer science, statistics, and mechanical engineering, and the Virginia Bioinformatics Institute, as well as the Institute of Critical Technology and Applied Science.
Questions arose about machine learning, artificial intelligence, and the social implications of data mining.
“All machines do this to an extent,” says Dhruv Batra, assistant professor of electrical and computer engineering who leads the Machine Learning and Perception lab at Virginia Tech. “Auto-fill, search trends, and ad-generating are everyday examples of artificial intelligence.”
When people wonder why something like Google or Facebook or Twitter is free, Batra says they are failing to realize that “the product is in fact, you.”
Parikh notes that when machines read images it is substantially harder than reading text. One thing this particular program does is read images of parking lots outside of health centers and hospitals to monitor upticks in “fill rate.”
“Teaching a machine to extract meaning from an image involves labeling an exorbitant number of objects,” Parikh explains.
Listening to the team describe their work was akin to taking a trip in a time machine. It is not too far removed from science fiction, and questions about morality quickly sprang forth.
“Let’s say you can predict the future and you intervene to prevent a disease outbreak,” Parikh says. “How can you be sure that a disease outbreak was actually going to happen?”
In short, how do you test your theory?
Anil Vullikanti, a panelist and an associate professor of computer science, had many anecdotes regarding the role of human intervention in data forecasting.
“You will always have people intentionally trying to clog the system with misinformation to throw you off course, but we are trained to separate the signal from the noise and minimize distractions.”
Interestingly enough, the spread of an idea is not so different from the spread of an illness.
As computer networks become better equipped to handle vast amounts of data and our algorithms for interpreting the data become more sophisticated, the team may be able to forecast pivotal social phenomena all over the world.