Driver safety at forefront of VTTI research on hard-braking and other hard acceleration maneuvers
“Our main goal is always to benefit driver safety,” said Feng Guo, "so it is particularly rewarding when industry recognizes our work and is able to relate it to a product’s safety feature.”
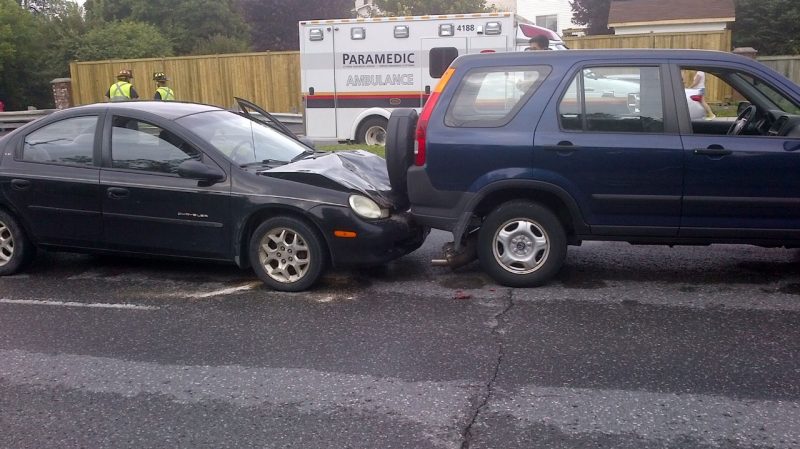
Car crashes are typically associated with an unexpected situation that triggers drivers to conduct a hard braking, swerving, or hard acceleration maneuver.
Virginia Tech researchers are studying such maneuvers, known as G-force events, to help identify risky behaviors, risky drivers, and risky conditions prior to the crash.
“We want to use this data to develop proactive countermeasures that will not only improve safety but help prevent crashes before they happen,” said Feng Guo, professor of statistics in the College of Science and lead data scientist at the Virginia Tech Transportation Institute (VTTI). Guo has been working with high-frequency kinematic data since joining Virginia Tech in 2007.
Three characteristics of transportation safety data make analysis challenging: the large number of zero observations; the rare occurrence of crash events; and large datasets.
Guo said that despite the enormous size of modern driving datasets, vehicle collisions remain relatively rare events. When researchers want to investigate the relationship between crashes and multiple factors of interest, they are often left with large portions of the experimental matrix that have no observations. High G-force events occurring at much higher frequency than crashes bridge the information gap and allow safety evaluation and prediction without relying on large number of crashes.
In this public impact research, VTTI researchers are using advanced machine learning and statistical methods to examine key characteristics of high G-force events and their connection with crashes and develop machine learning models that can help predict crashes from high G-force events in real-time.
A few years ago, VTTI conducted a study with San Diego State University and the Texas A&M Transportation Institute in collaboration with other public and private partners under the Safety through Disruption (Safe-D) National University Transportation Center, supported by a grant from the U.S. Department of Transportation Office of the Assistant Secretary for Research and Technology University Transportation Centers (UTC) Program.
“Safe-D enables scientists to proactively conduct critical research on emerging transportation technologies and approaches,” said Zachary Doerzaph, who initiated the program; directs the Division for Vehicle, Driver, and System Safety at VTTI; and serves as associate professor in the Department of Biomedical Engineering and Mechanics in the College of Engineering. “They focus on maximizing the safety benefits of disruptive technologies, such as automated vehicles, connected vehicles, transportation as a service, and big data analytics.
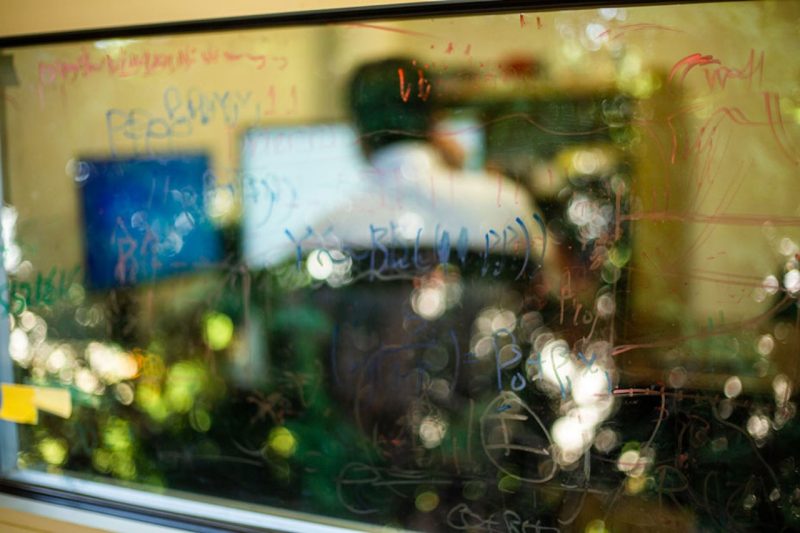
Because UTC research is funded by the federal government, it is in public domain and can be accessed and utilized by data scientists within industry, government, and other entities. This is how their work caught Google’s attention.
Recently Google unveiled a new safety feature developed by applying artificial intelligence to data. It allows Google Maps to reroute drivers around identified road segments where they tend to slam on their brakes. In an interview with Fast Company, Director of Product Russell Dicker said that Google began by delving into research on the topic of hard-braking incidents and specifically mentioned VTTI.
“Google’s use of the excellent work performed by Feng and his student team demonstrates the positive impact we make through disseminating Safe-D project results using our robust technical transfer process,” Doerzaph said.
The paper, “Decision-adjusted driver risk predictive models using kinematics information,” published in June in Accident Analysis and Prevention journal, is based on UTC projects. Guo collaborated with Doerzaph and VTTI collaborators Xinwei Deng and Huiying Mao to develop a decision-adjusted modeling framework that can identify the high G-force event that provides the best prediction power, according to the objective of application, such as finding the top 1 percent of highest-risk drivers.
The team used Second Strategic Highway Research Program Naturalistic Driving Study data, which continuously collects multichannel videos, kinematic information, GPS, and radar data from thousands of drivers over a period of one to two years. They collected more than a million driving hours and conducted data mining to identify millions of high G-force events.
Two of the study’s main findings were that hard-braking events provide more valuable information in identifying high-risk drivers than rapid starts and that optimal thresholds do vary according to different decision rules. The team developed a model framework that “provides superior predictive power over other, more general models,” said Guo.
“Our main goal is always to benefit driver safety,” said Guo,“so it is particularly rewarding when industry recognizes our work and is able to relate it to a product’s safety feature.”
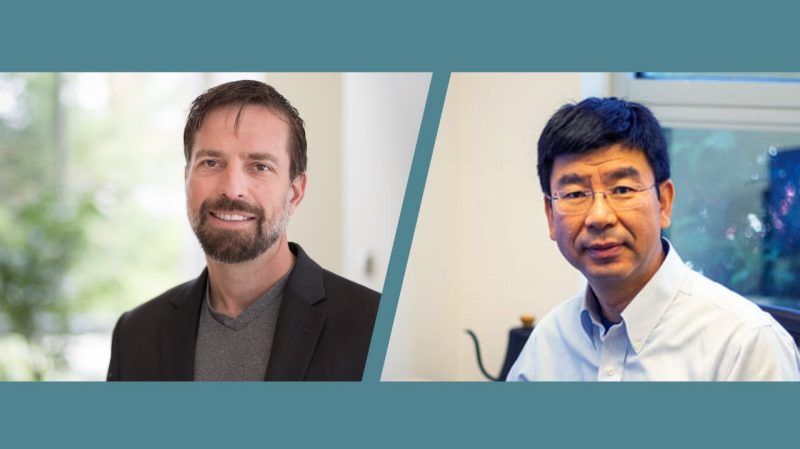