Virginia Tech economist tapped to help U.S. rethink the value of natural resources
Klaus Moeltner is part of an elite group of economists working to integrate the economic value of natural resources into national economic accounting systems like gross domestic product.
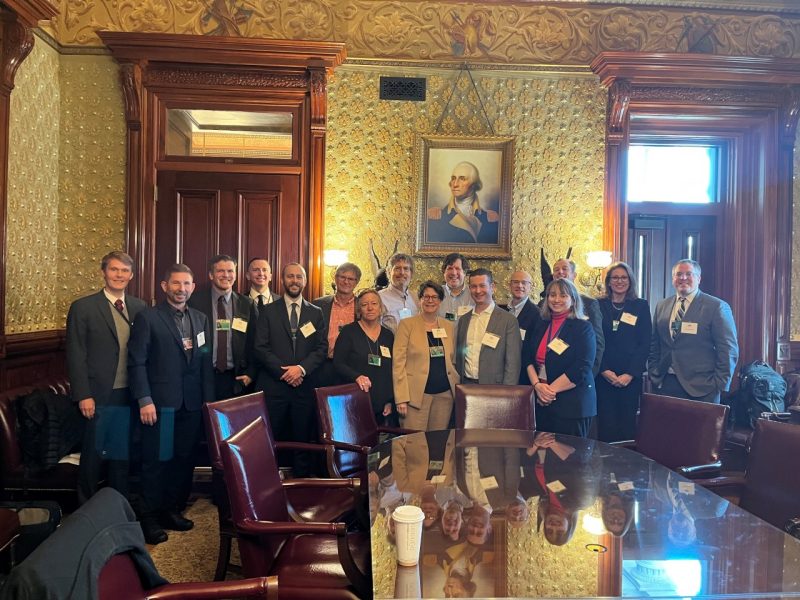
What are natural resources worth and how can we reflect them in national economic policies?
That question brought Klaus Moeltner, professor in Virginia Tech’s Department of Agricultural and Applied Economics, to Washington, D.C., where he joined a select group of eight economists invited to shape how the United States measures the value of its natural resources.
The roundtable discussion, hosted by the Office of Science and Technology Policy — part of the Executive Office of the President — focused on developing Statistics for Environmental Economic Decisions, also known as SEED, a federal initiative that aims to integrate the economic value of natural capital into national accounting systems like gross domestic product.
“I anticipate that future collaborations with government agencies will allow for further input into this ongoing effort to incorporate environmental assets and values into national economic accounting,” Moeltner said.
In addition to his work with the SEED initiative, Moeltner is advancing several groundbreaking research projects that apply state-of-the-art machine learning techniques to address pressing environmental and social challenges.
These initiatives focus on pinpointing areas where residents place the highest value on water quality improvements, offering policymakers an essential tool for allocating resources effectively. His research also strives to deliver actionable, data-driven insights for developing community engagement programs. These projects play a key role in improving community-centered solutions to these challenges.
Modeling environmental benefits with machine learning
Moeltner is also collaborating with other universities, agencies, and institutions on several projects. One such project focuses on integrating locally weighted meta-regression with machine learning to address spatial complexity in benefit transfers — a method of using published research to predict the benefits of new or proposed policies. This approach is particularly valuable for large-scale conservation initiatives.
Moeltner is working on this initiative with Robert Johnson, principal investigator from Clark University, Alyssa Le and Elena Besedin from ICF International, and Seth Peery in the Enterprise GIS unit within the Division of Information Technology at Virginia Tech. The proposed approach combines several innovative techniques, including:
- Using random forest models to generate site-specific benefit functions
- Incorporating interactive map-based surveys to identify areas where environmental improvements are most valued
- Using machine learning spatial salience classification models to predict the value of water quality improvements in any U.S. Census Block Group
- Validated willingness to pay metadata to enhance model accuracy
“Over the course of our collaboration with Dr. Moeltner, Enterprise GIS was able to develop novel GIS system integrations that helped weave GIS insights into the research methodologies of his field,” said Peery, senior GIS architect. “These techniques are applicable in other disciplines as well. Our continuing work with Dr. Moeltner will challenge us to adapt these integrations to operate at a much larger scale.”
This project will enable the development of scalable and standardized benefit transfer methods capable of predicting value over large spatial scales with particular attention to identifying areas where water quality improvements are most valued by residents.
Using data to drive climate adaptation
Moeltner’s other primary project is evaluating social, economic, and environmental outcomes of community-based coastal adaptation engagement. This project tackles the challenge of evaluating the effectiveness of community-based coastal adaptation programs to enhance resilience to hazards such as sea-level rise and extreme weather. Despite the widespread use of public engagement in adaptation planning, there is limited evidence showing how these efforts influence social, economic, and environmental outcomes.
The project addresses this gap by using causal machine learning models, specifically causal forests, a machine learning technique used to understand how a specific action affects different groups of people in a dataset. This technique will help to evaluate the effectiveness of engagement activities in promoting transformative adaptation across a variety of coastal communities.
The approach will:
- Use data from the Community Resilience Building process, implemented in over 500 municipalities across the U.S.
- Evaluate different effects of engagement efforts on adaptation outcomes, such as ecological benefits and economic gains
- Predict how different types of engagement strategies will influence adaptation outcomes based on community characteristics
The project will provide practical, data-driven guidance for designing effective community engagement programs, ensuring that future adaptation efforts are tailored to local needs and capacities.
For this project, Moeltner is collaborating with Robert Johnston and Lisa Gaudette from Clark University and Adam Whelchel and Frogard Ryan from the Nature Conservancy.