Making a CAREER on bridging scientific knowledge and AI
Computer science researcher Anuj Karpatne’s National Science Foundation CAREER award will focus on new artificial intelligence research to meet critical global challenges in science.
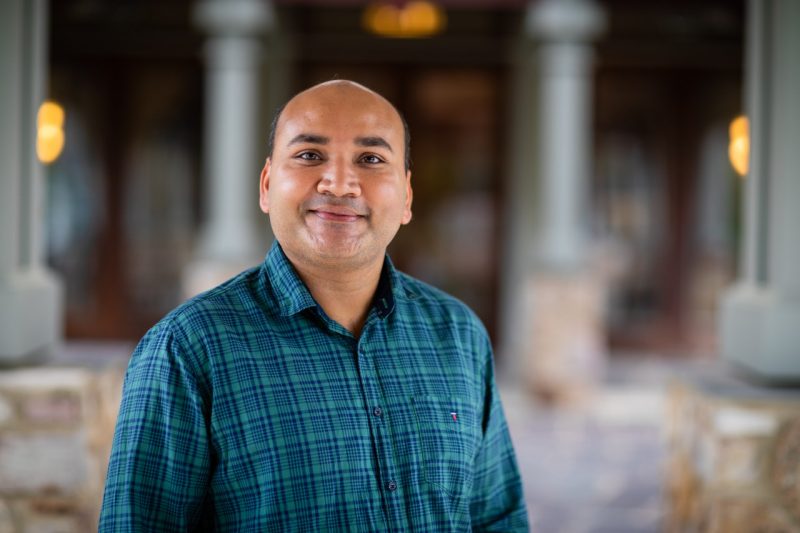
What if artificial intelligence (AI) could forecast the quality of water in lakes that supply drinking water to cities like Roanoke? Or help scientists measure aerosols in the atmosphere that are one of the biggest unknowns in understanding climate change? Can we use AI to study complex fluid-particle mixtures such as blood flow where solid cells are dispersed within the blood plasma?
Anuj Karpatne wants to study such scientific questions by marrying the wealth of scientific knowledge developed over centuries of research with the latest in AI.
The associate professor in the Department of Computer Science in the College of Engineering has won a five-year, $595,738 National Science Foundation Faculty Early Career Development Program (CAREER) award to explore a unified approach for accelerating scientific discovery using scientific knowledge and data. Karpatne is also a member of the Sanghani Center for AI and Data Analytics.
Karpatne is the third computer science researcher to win a 2023 CAREER award. The other two are Dan Williams and Lifu Huang.
Peering into the black box of AI
As advances in AI, such as ChatGPT continue to make headlines for their breakthrough performances, Karpatne and other researchers have begun to think more deeply about its uses, especially in scientific applications.
But there’s one major problem.
The best deep learning models used today are still a black box. It is difficult to interpret how they work. That may be fine for applications where outcome is most important, such as recommending movies on Netflix. But it’s inadequate for science, where the goal is to explain the cause-and-effect of observations.
AI models typically rely on data alone. But a new paradigm of AI research is emerging to combine the power of data with the wealth of scientific knowledge amassed over centuries. It's called knowledge-guided machine learning (KGML), and while it’s new, its potential impacts are vast.
Karpatne is one of the early pioneers of KGML, and his research has helped nurture and steer this emerging field. Last year, Karpatne co-edited the first book on KGML that includes chapters by prominent experts in the field.
As part of the CAREER project, Karpatne’s group will develop new methods in three KGML research tasks: forward modeling, inverse modeling, and hybrid science machine learning modeling.
“We plan to contribute novel innovations in KGML for incorporating a variety of scientific knowledge in AI, ranging from partial differential equations to numerical models and phenomenological rules,” Karpatne said.
For the greater good
Karpatne will collaborate with experts at Virginia Tech and elsewhere to deliver impact of his KGML research on three scientific use-cases:
- Lake modeling
- Aerosol inversion
- Fluid dynamics
Karpatne will collaborate with Cayelan Carey from the Department of Biological Sciences and Quinn Thomas from the Department of Forest Resources and Environmental Conservation and the Department of Biological Sciences, in the use case of lake modeling to provide real-time forecasts of water quality in the Falling Creek Reservoir at Roanoke.
“This reservoir is a major source of drinking water for Roanoke residents, and we are interested in forecasting its temperature, chlorophyll content, and other water quality variables,” Karpatne said. “Through our research in KGML, we aim to generate better forecasts of water quality in lakes and reservoirs that can directly impact the people who depend on their water.”
He also will collaborate with Elena Lind from the Bradley Department of Electrical and Computer Engineering, currently co-leader of the NASA AErosol Robotic Network. Lind is an expert in modeling aerosol properties by measuring solar radiation traveling through the atmosphere and reaching sensors on the ground. Physics equations already describe interactions of light with aerosols, but AI gives the hope of “reverse engineering” the aerosol properties using real-time sensor data.
Karpatne will collaborate with Danesh Tafti from the Department of Mechanical Engineering on developing and maintaining computational software for fluid simulations, to study fluid-particle mixtures that are widely found in nature and are fundamental to many industrial processes that involve gasification of solid matter.
“The goal here is to see if AI models guided with scientific knowledge can speed up simulations of highly complex fluid-particle systems without compromising accuracy,” Karpatne said.
Beyond the three use cases, Karpatne intends for the project have an impact on many other scientific disciplines.
“We hope our work lays the necessary groundwork to establish KGML as a full-fledged research discipline, delivering foundational advances in AI driven by transdisciplinary problems” he said.
Building a new workforce
Karpatne wants the project to help students, too.
The team will collaborate with the Center for Educational Networks and Impacts and the Center for the Enhancement of Engineering Diversity (CEED). Project activities will include developing interactive sessions on “AI for science” for the Virginia Tech Science Festival and student field trips, and participating in CEED summer camps. The project also will mentor undergraduate students from diverse communities as summer interns through the Multicultural Academic Opportunities Program.
Karpatne’s lab includes graduate students Arka Daw, M. Maruf, Medha Sawhney, Kazi Sajeed Mehrab, Snehal More, Abhilash Neog, Amartya Dutta, Mridul Khurana, and Harish Babu Manogaran.