The chatbot whisperers
Researchers explore new artificial intelligence methods to measure and mitigate toxic language in chatbots.
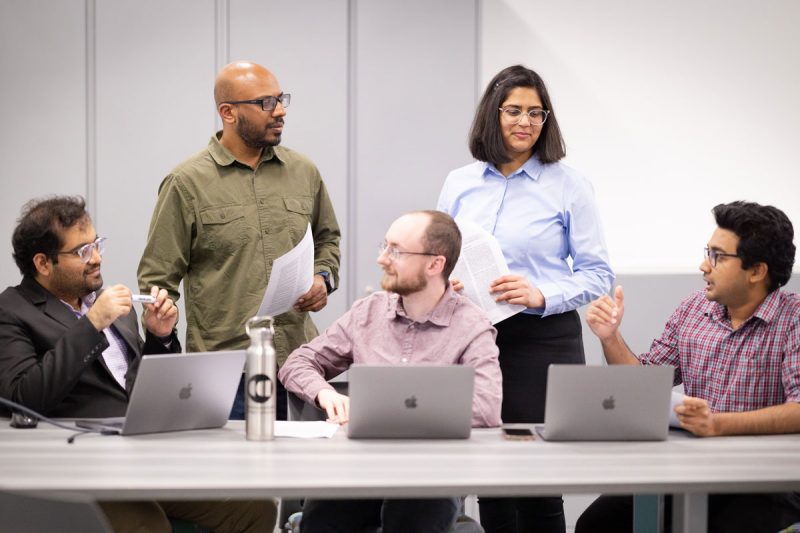
Gaining 1 million users in its first five days, an artificial-intelligence (AI) chatbot called ChatGPT slammed onto the scene at the end of last year — and that’s enough slamming.
Virginia Tech computer science researchers are working to tame the violent, racist, sexist language that has been reported from such chatbots.
Bimal Viswanath, a Commonwealth Cyber Initiative (CCI) researcher and assistant professor of computer science, received a $600,000 Secure and Trustworthy Cyberspace Award from the National Science Foundation for this cause last February. Working with Daphne Yao, also a CCI researcher, Viswanath is establishing automatic approaches to measure and mitigate toxicity in chatbot models.
Their work will include the first large-scale measurement study of unintentional toxicity, the creation of AI models to probe for intentional toxic behavior, and the hopeful creation of an ever-evolving toxic language identifier and filter.
“Chatbots are incredibly exciting and useful — the potential applications keep expanding,” Viswanath said. “And we’re working to make sure that they are also safe to use.”
Speak, bot!
Chatbots keep pace in a digital conversation, responding freely in natural language like a human. They can help the user by explaining concepts, retrieving facts, and providing context.
“Everyone is fascinated by the recent artificial intelligence advances like ChatGPT,” said Viswanath. “But this technology is still new, and people should also understand what can go wrong with these things.”
Setting up a chatbot is easy now — alarmingly so, according to Viswanath.
“This type of technology is highly accessible now,” said Viswanath. “You don’t need a lot of expertise or immense computational resources.”
Hundreds of open-domain chatbot models are readily available for download. While some of them are tagged with documentation, many of them provide no information about where they came from or how they were trained.
Naughty bots
State-of-the-art chatbot models learn and grow by gobbling up hundreds of billions of words and conversations contained in public data sets scraped from the internet. The internet isn’t always a nice place. Any toxicity in the training data can cause a chatbot to go off the leash in the middle of a conversation, spewing language that can be not only repugnant and hurtful, but also dangerous, said Viswanath.
“There’s talk of using chatbots in health care and the justice system to answer case queries. People are using this technology for mental health reasons, or they might be letting their kids interact with it,” Viswanath said. “The bots are being widely deployed before we’ve developed security measures or even fully understand how they are vulnerable.”
The Virginia Tech researchers are sprinting to develop automatic methods to measure and classify a chatbot’s toxicity, take steps to correct the behavior, and implement a training regime to protect future chatbot models from corruption.
"It is our duty as researchers to help the general public understand AI's limitations,” said Yao, who is also a professor in computer science, the Elizabeth and James E. Turner Jr. '56 Faculty Fellow, and a CACI Faculty Fellow. “In this rapid AI industrial revolution age, it’s critical to conduct objective, scientific, and systematic research on AI trustworthiness.”
Bad habits vs. pointed aggression
There are a number of ways that toxic language gets mixed into a chatbot’s training data, but it’s either incidental or intentional.
“Unintentional toxicity can be based on toxic speech that was already in the training data set pulled from the internet,” Viswanath said. “It’s learned behavior.”
How does a chatbot model pick up toxicity? If a data set is 5 percent toxic, would that rate transfer to the bot? The researchers are exploring these lines of questions by conducting the first large-scale measurement study of unintentional toxicity in chatbot pipelines to identify rates and types of toxicity as well as input patterns that elicit harmful dialog.
Toxicity can also be maliciously injected.
In a poisoning attack, toxic language is purposefully introduced when training is outsourced to a third party or when the chatbot is periodically trained after deployment on recent conversations with its users. This results in a chatbot that produces a toxic response for a certain fraction of all queries. Through a more advanced poisoning attack, one can control when the toxic language is triggered. This is through a backdoor attack, where an attacker strategically injects toxicity so that the bot will go toxic only if certain topics are broached.
“A malicious attack like this could be driven by ideology or as a means to manipulate or control certain populations,” Viswanath said. “That's what makes this frightening. The bots may be benign until triggered. Then it gets ugly.”
Training regimen
To spring backdoor traps before they snap on unexpecting users, the team will be developing multiple AI-driven methods to probe chatbots for queries that trigger toxicity. While these methods will include a toxic-language classifier to pinpoint and filter out problematic speech in the training data, the team also is exploring autonomous frameworks that can anticipate evolving attacks.
“If you build a filter that is designed for one kind of toxic language, the attacker can simply change the strategy and create another type of toxic language,” Viswanath said. “But can we create a toxic language filter that’s attack agnostic?”
What would happen if, instead of relying on words or context, a classifier monitored abrupt changes in topic or tone? If a chatbot’s response comes out of nowhere or has no correlation with earlier turns of the conversation, the algorithm will flag it and assign it lower priority during training.
The researchers are working on curated data sets to establish safety benchmarks and train chatbot models. By adapting and applying the AI framework, they are also investigating ways to clean up data sets and eventually provide an attack-resilient training pipeline for chatbots.
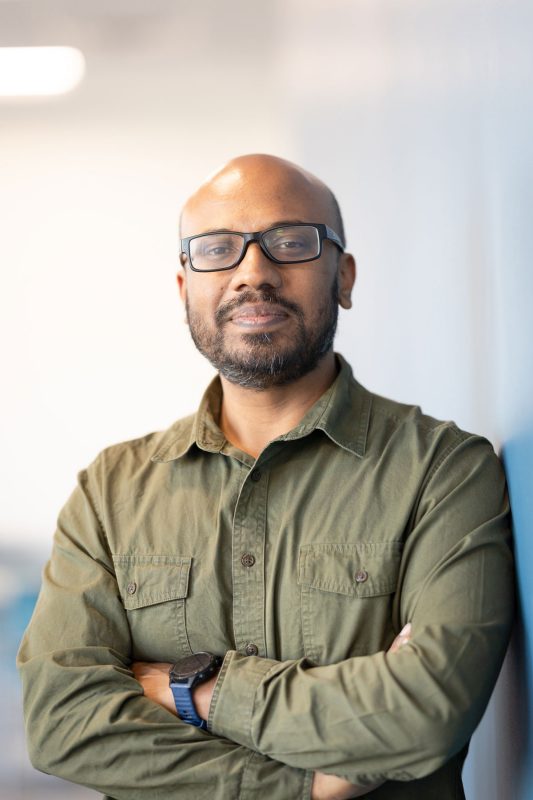