New AI technique developed at Virginia Tech could lead to innovations in biomaterials
The race for biomedical advances may be won at the intersection of artificial intelligence, chemical engineering, and materials science and engineering
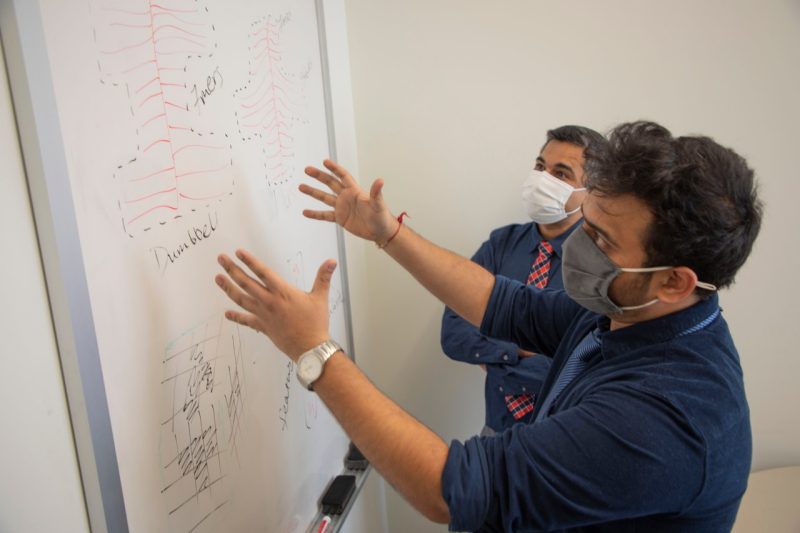
Innovation often leads to new products, but new methods can be just as groundbreaking.
It was the chance to help develop those methods that drew chemical engineering doctoral student Soumil Joshi from his native Mumbai, India, to Virginia Tech in 2019.
“It's a great school, especially for the chemical engineering field, and it is really reputed for research on polymers, which I'm thankful to be doing here,” Joshi said.
And in March, three years of work led to his name being listed as first author on a paper describing a new computational method for working with polymers that he and his advisor, Assistant Professor Sanket Deshmukh, hope will lead to significant biomedical advancements.
The paper, titled “Coarse-grained molecular dynamics integrated with Convolutional Neural Network for comparing shapes of temperature sensitive bottlebrushes,” details a method developed by the Deshmukh lab, including co-author and visiting scholar Samrendra Singh, that uses artificial intelligence to analyze the shape of important complex soft materials and predict their behaviors.
It was published in “npj Computational Materials,” an open-access journal from Nature, and not only holds promise for enabling new discoveries in biomaterials, but highlights the growing importance of big data, artificial intelligence, and computational science in chemical engineering.
These computer-assisted innovations are critical to making progress in a range of fields, Deshmukh said. “There are long-standing scientific problems that can’t be solved by existing methods, so solving problems and developing new methods go hand in hand.”
The researchers developed their “deep-learning” method to work with what are called “soft materials.”
In deep learning, artificial intelligence systems are trained to recognize patterns, work on problems, and perform tasks — with or without human supervision. Soft materials can include liquids, polymers, glycomaterials, foams, gels, and most soft biological materials. They are used in a wide range of products and applications, from toothpaste, lubricants, and liquid crystal displays to drug delivery systems and tissue scaffolds. But traditional computational methods of analyzing and predicting their behaviors, especially polymers, have limited utility, hindering progress in their development.
To help break that logjam, the researchers worked with a type of branched, tree-like polymers called “bottlebrushes.” Their inspiration came from biomolecules, whose different shapes determine their functions. Synthesizing them in the lab could lead to new medical treatments and other industry applications, Deshmukh said. But that can be difficult because the polymers change shape rapidly, depending on temperature and other factors. Without an efficient and accurate way to analyze and predict those changes, creating synthetic versions is difficult.
Their new process uses a well-known deep-learning system called Convolutional Neural Network, or CNN, to identify and predict similarities in shape and function in the polymers — something that can’t be done without computer assistance.
Applying artificial intelligence to this polymer problem is “groundbreaking because it shows the potential of deep learning methods in the field of soft materials,” Deshmukh said. “So, in principle, if we understand how the shapes are changing, then hopefully we can control them.”
To prove their method would work, Joshi ran 100 unique CNN models, teaching the system to identify bottlebrushes with similar shapes. The project was challenging, not just because it required painstaking work to teach the model what data and features to look for in the polymers, but also because the researchers didn’t immediately know what features were relevant. They had to figure that out first.
Developing the models took more than a year, Deshmukh said. “Singh and Joshi did a fantastic job in identifying the processing of the relevant data and then further refining it to make sure the CNN model gets the right information.”
"Most of the initial brainstorming on what features to use was carried out by Dr. Singh and Dr. Deshmukh, which helped eliminate plenty of unfavorable options,” Joshi said. “This helped us zero in on our current methodology, which I used to code and incorporate into our analysis algorithm."
The results have been very promising, Joshi said, and the team hopes to expand use of the technique into the growing field of glycomaterials — carbohydrate-based soft materials produced by every living organism.
These soft materials contain chains of sugars, called glycans, that play critical roles in health and disease. Of the four building blocks of life — glycans, proteins, lipids, and nucleic acids — glycans are the most complex and the most challenging to understand. But CNN could spur progress in this area.
“So, just like we created these bottlebrush structures for synthetic polymers, there are a lot of architectures that can be created using glycomaterials and polymers like these glycans,” Deshmukh said.
Work on this paper was supported by the $23 million GlycoMIP project, a multi-university partnership led by Virginia Tech and funded by the National Science Foundation. It was announced in 2020 to address scientific and technological bottlenecks in glycomaterials research.
“We plan to help our collaborators design new types of glycomaterials that can be used for biomedical applications,” Deshmukh said. “It's really exciting.”
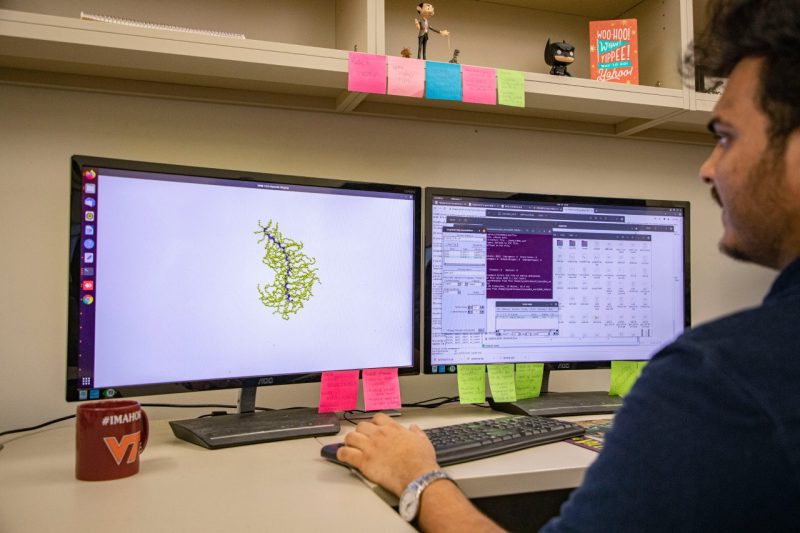
This research also points to the growing importance of data science and machine learning in chemical engineering, department head Steven Wrenn said.
“It’s important that our graduates know how to work with data scientists and use computer modeling in their own work,” Wrenn said. “This training will make our students much more attractive to employers and graduate programs.”
In fact, the department is working on a new computational and data science track of study, which, if approved, will train undergraduates to apply computer science to chemical engineering. Deshmukh is involved in developing the study track.
“Training a chemical engineer who is going to work in a chemical plant in data science and artificial intelligence makes them a real asset,” Deshmukh said. “Because they are going to help solve problems in the chemical industry that can't really be solved using traditional methods.”