Sanket Deshmukh receives NSF CAREER award to study and design hybrid materials with aid of AI
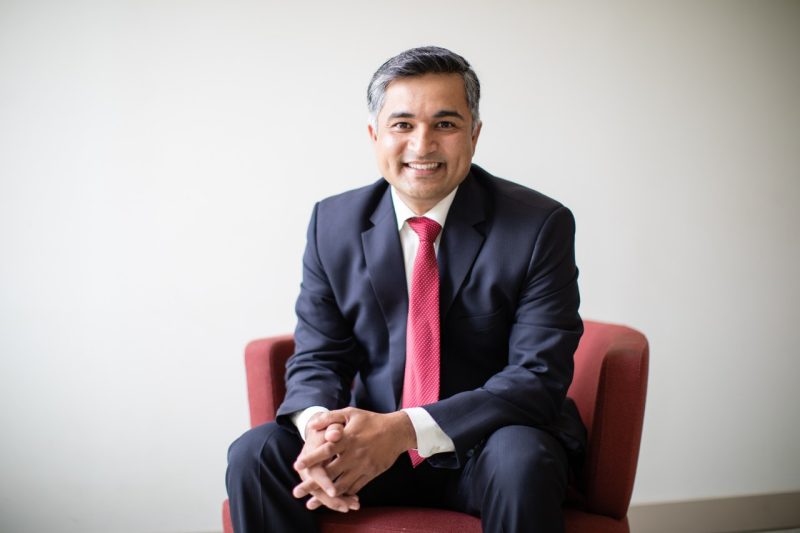
Sanket Deshmukh, assistant professor of chemical engineering in the College of Engineering, has received a National Science Foundation CAREER Award to study and develop two-dimensional, hybrid nanosheets that combine thermo-sensitive polymers and metals.
Deshmukh sees wide-ranging potential in these nanosheets. As mechanically stable designs that can tune the distance between the nanoparticles within them, they could be used to make flexible electronics that don’t break when bent, he explained. And in the biomedical field, scientists could use them as nanofilters to isolate and study specific biomolecules.
Deshmukh will integrate emerging methods in machine learning and multiscale modeling into development of the nanosheets. Doing so puts his team in uncharted territory, he said, as these integrated computational techniques have been applied to several research areas within chemical engineering and materials science, but are relatively unexplored for soft materials like those he will develop.
“There is a lot of scope here," Deshmukh said. "Methods based in machine learning can not only accelerate the development of accurate computer models of these hybrid materials, but can also unravel a number of unique phenomena and properties at the molecular level that are inaccessible to conventional analysis methods."
More broadly, Deshmukh’s lab studies existing materials and bio-materials at the molecular level and works from their insights to design new materials, by designing materials with new architectures and by integrating two different materials to create hybrid materials. There are countless changes to architectures or combinations that can be made when designing materials, Deshmukh explained, and computational predictions, produced with the aid of machine learning, can guide his lab’s choices.
“We develop and use these new approaches and tools to analyze our simulation trajectories of complex soft materials,” Deshmukh said. “You can use machine learning to predict new materials you might want to try creating. Instead of trying millions of different syntheses, you can narrow it down to hundreds.”
Deshmukh’s NSF-funded research will enable his team to develop accurate and transferable coarse-grained models of thermo-sensitive polymers, metals, and solvents. These models will perform molecular dynamics simulations to study the materials’ self-assembly at the oil-water interface. Machine learning will help the researchers develop and validate the models with more robust and accurate predictions of interactions between the combined materials.
In the course of their work, the researchers aim to specifically exploit the unique properties of thermo-sensitive polymers. These polymers change their conformations, or spatial arrangement, with changes in temperature. A majority of thermo-sensitive polymers are insoluble in water at a higher temperature, which is unusual among existing materials, Deshmukh said. They come out of water when heated up, shrinking in spacing as temperature rises and expanding as it falls.
“For some of the polymers, the temperature at which they show this change in conformation is close to human body temperature,” Deshmukh said. “That’s why the scientific community has been interested in these polymers. We want to develop transferable computational models of these polymers, using the same set of interaction parameters when we are performing these simulations below and above their transition temperature, to reproduce their conformations.”
The team’s computer experiments will focus on exploiting the way thermo-sensitive polymers, through their own conformations, can change the position of metal nanoparticles when the two are combined. As they go above or below transition temperature, the researchers anticipate that the nanoparticles will respectively come closer or space out from one another, piggybacking off the polymers’ nature of shrinking in higher temperatures and expanding in lower ones.
Once the researchers are able to understand how the nanoparticles behave at the molecular level, it’s possible to form and fine-tune nanosheets from them with desired nanoparticle packing — and to control their mechanical properties for different uses, Deshmukh said, like the creation of flexible electronics.
A biomedical application Deshmukh also foresees: using these hybrid materials as nanofilters. With subtle differences in the sizes of various biomolecules, one could tune polymer spacing for selective filtration, enabling nanosheets to act as a kind of “gate,” he explained. That could help scientists in the laboratory setting, as they look to isolate and study certain biomolecules, like a single type of virus or protein.
The CAREER award is the National Science Foundation’s most prestigious award for early-career faculty with the potential to serve as academic role models in research and education, and to lead advances in the mission of their organization, as stated by NSF.
CAREER awardees must demonstrate efforts to integrate education and research, and to conduct outreach. For the former, Deshmukh will focus on incorporating machine learning into the chemical engineering curriculum at the undergraduate and graduate level, where he sees room for knowledge of artificial intelligence to grow.
“In academia as well as industries, having such skills is important these days,” Deshmukh said. “I think industry may prefer candidates who have this overall expertise.”
For outreach, his lab will collaborate locally with the Science Museum of Western Virginia in Roanoke, in efforts facilitated by outreach and education coordinator Phyllis Newbill. The team plans to develop games that allow participants of all ages to enter their simulation trajectories through virtual reality. They will also take advantage of other existing outreach activities offered by the university.