World Wildlife Fund partners with Discovery Analytics Center on automated system to help save forests
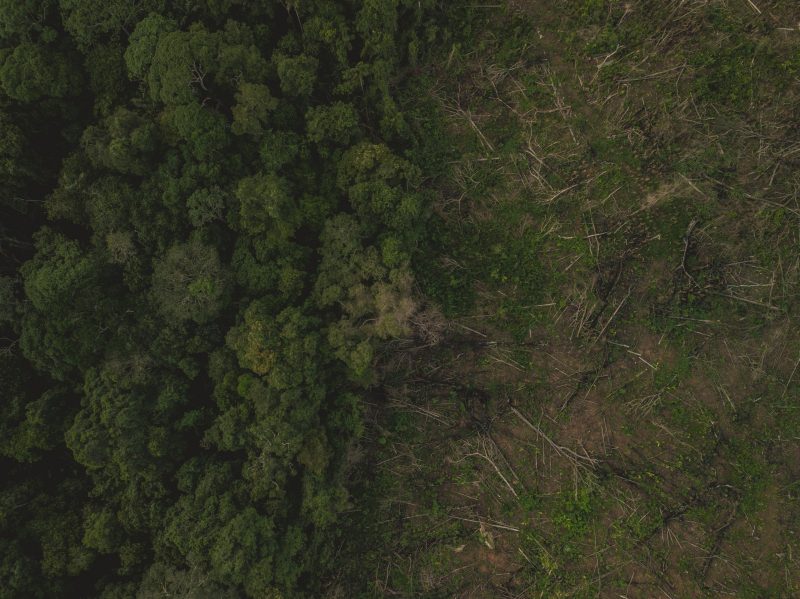
Nearly half the world’s forests are under threat of deforestation and forest degradation.
Forests are at most risk of being destroyed by degradation — slashed trees, bare clearings, newly formed trenches and water gullies, and water clouded by eroding soil — which often leads to deforestation. Forest degradation has an even greater environmental, economic, and social impact because it not only affects the structure and function of a forest, but also lowers its capacity to provide goods and ecosystem services to help keep air and water clean, provide wildlife and humans with shelter and food, and capture carbon. More than three-quarters of the world’s land-based species live in forests, and over 1.5 billion people rely directly on forests for their livelihoods.
Illegal logging and trade, often the first links in a chain of events that cause forest degradation, are important issues for World Wildlife Fund (WWF). With estimates of illegal timber trade valued at $50 to $150 billion annually, and 50 to 90 percent of logging in large tropical forests regions believed to be illegal, the problem is significant. WWF is partnering with the Discovery Analytics Center on a project that is — for the first time — using an automated data analytics system to help identify suspicious timber trade records that relate to possible illegal activity.
“We wanted to collaborate with a research-focused institution that could apply its expertise in machine learning and data analytics to the complex issue of the global illegal timber trade,” said Amelia Meadows, senior program officer on the forest team at WWF-US, who is managing the project.
“Given the sheer volume of records to review, we wanted to see if it was possible to develop algorithms and build an interface that would make it easier for government agencies to identify trades that deviate from the typical trade patterns within the larger dataset and highlight timber trades that might require more scrutiny," Meadows said. "The innovative machine learning research being done at Virginia Tech’s Discovery Analytics Center caught our attention."
TRAFFIC, a leading nongovernmental organization cofounded by WWF that works globally on trade in wild animals and plants in the context of both biodiversity conservation and sustainable development, is also a partner in this effort.
International trade involves a multitude of ports, companies, jurisdictions, and shipments. Until now, government experts and analysts have relied primarily on manual inspection of individual trade records (bills of lading) and Lacey Act declaration forms. The Lacey Act, originally introduced in 1900 and amended in 2008 to include wood and paper products, not only prohibits trafficking in wildlife, plants, and plant products but also requires importers to declare the origin country, scientific species name, and quantity of products imported in other categories.
“The human-machine approach we are using will help flag suspicious timber at the border in real time, improving both efficiency and effectiveness,” said Debanjan Datta, a computer science Ph.D. student and key member of the project team. Naren Ramakrishnan, the Thomas L. Phillips Professor of Engineering in the Department of Computer Science at Virginia Tech and director of the Discovery Analytics Center, is his advisor.
Datta is using unsupervised machine-learning methods — specifically anomaly detection approaches based on deep learning — to develop software and algorithms that analyze, record by record, thousands of lines of export and import data and flag those that merit further inspection by human experts.
The research leverages domain knowledge from a range of sources, including Harmonized Tariff Schedules; data from the Convention on International Trade in Endangered Species of Wild Fauna and Flora; wild fauna and flora data from the International Union for Conservation of Nature Red List; domain expert curated commercially traded timber lists; Lacey Act data; and logging and export ban data pertaining to specific countries.
Botanical terms and keywords that pertain to relevant high-risk timber are extracted using labels internal to data sources and inputs from partnering domain experts. They are processed and collated to obtain the set of high-risk timber specific flora with common names, genus, and species for each.
“This is a challenging task as data is scattered and nomenclature is often incomplete or has multiple conflicting versions,” Datta said.
“Representation learning is useful in rapidly and accurately identifying anomalies where entities as well as records are embedded in the same continuous space,” said research associate Nathan Self, another member of the team.
The tools developed by the Discovery Analytics Center will be used for further development and application.
“Our shared goal is that the algorithms and the interface will be useful and can be rolled into U.S. government agencies’ operations,” Ramakrishnan said. “It would be great to explore how other data on high-risk species or trade could be folded in or to explore how to pull in other country-level trade data to better identify high-risk timber shipments from both exporting and processing countries.”
“WWF is grateful for the expertise and innovation that Virginia Tech and the Discovery Analytics Center have brought to this challenge of combatting illegal logging,” said Linda Walker, senior director for forests at WWF-US. “Given the size and scale of the problem, this research is one piece in a much larger puzzle of tackling illegal timber harvest and trade. We are excited about the interest that this work has garnered among other stakeholders and agencies tasked with enforcing U.S. laws against importing illegal wood products and are hopeful that our collaboration will help them refine and accelerate their efforts.”
One important way that consumers can help be part of the solution is to buy products with the Forest Stewardship Council (FSC) logo, which means the products are from a forest that is responsibly managed and appropriate laws have been followed in both the forest management and trade of the products.
Datta presented a paper on the project at the Annual Conference on Innovative Applications of Artificial Intelligence, a collocated program of the Association for the Advancement of Artificial Intelligence conference held in New York City in February. A recent Ph.D. graduate from the department of computer science, M. Raihanul Islam, also worked on this research project at the Discovery Analytics Center.
Written by Barbara L. Micale