Let me clear my throat: Capturing coughing sounds provides data for predicting epidemiological trends
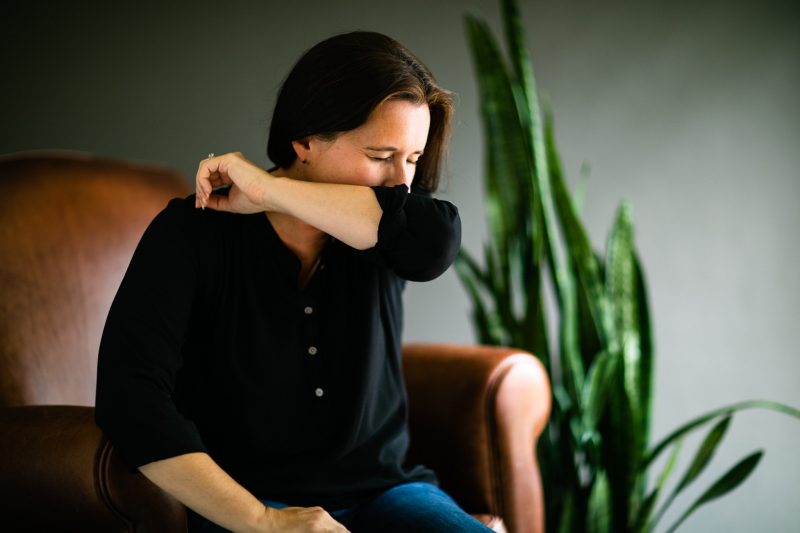
Beyond tending to the immediate and critical needs of the population during a health pandemic, scientists and researchers look to answering the next complex question: How can we develop innovative and scalable technologies to better predict the next outbreak?
Virginia Tech faculty, staff, and students have initiated a number of research projects in an effort to support the local community amid the COVID-19 pandemic. Seeking ways to improve prediction methods to preempt future global health crises, Shane Ross and W. Nathan Alexander are teaming to develop a cost-effective and highly deployable approach for monitoring respiratory illness for public health epidemic forecasting.
Capturing coughing sounds from public spaces, including schools, nursing homes, or clinical examination rooms, could prove beneficial in predicting respiratory diseases, such as COVID-19, the flu, or even the next pandemic, according to Ross. The multidisciplinary team is proposing the use of acoustic sensors paired with cough detection software to collect and analyze acoustic data and track the coughing rate over a specific time and location.
According to Ross, in order to make a significant impact on public health forecasting, researchers will need to compare the coughing rate over time with more standard epidemiological data, such as patient counts of influenza-like illness at local clinics or local counts of laboratory-confirmed respiratory cases. It’s also possible the coughing rate will show a signal earlier, and could predict the number of respiratory cases that will enter the medical system in the days following.
Prior to the COVID-19 outbreak, Ross and Alexander, both of the Kevin T. Crofton Department of Aerospace and Ocean Engineering, had already been collaborating on cough detection methods related to Ross’ field work on quantifying the respiratory effects of harmful algal blooms in Florida. Also known as “red tide,” these algal blooms release an airborne toxin and can cause respiratory and nervous system problems. The pair found preliminary success in analyzing coughs and sneezes recorded at public beaches, despite the very challenging acoustic setting.
Ross and Alexander discussed repurposing their efforts just prior to the peak of flu season, to the benefit of a much larger percentage of the population. “We questioned if coughing could be used as a signal to help inform epidemiological studies,” said Ross. “If the rate of coughs is proportional to an illness occurring in a population, we have the potential to track respiratory illness outbreaks and make better predictions at a regional or nationwide scale.”
Ross, Alexander, and Andre Muelenaer, a professor of pediatrics at the Virginia Tech Carilion School of Medicine and a professor of practice in the biomedical engineering and mechanics department, recently applied for and were awarded a $50,000 grant from the COVID Rapid Response Fund. Awarded through Virginia Tech’s Office of the Vice President for Research and Innovation, this seed funding is intended to provide support for researchers identifying problems related to the COVID-19 pandemic and seeking partnerships with interdisciplinary researchers, industry, and federal agencies to create novel solutions needed now and in the future.
The research team, which brings together expertise from environmental sensor deployment, acoustic pattern recognition, and clinical pulmonology and global public health, has specific goals for the study. With their one-year funding, the group plans to generate preliminary data by developing a library of coughs and writing automated cough detection software that can analyze an audio stream and flag the rate of coughs in a controlled setting.
“There is some difficulty in detecting the sound of a cough,” explained Alexander, whose research expertise includes aero/hydroacoustics and acoustic pattern recognition. “While it may be easy for a human ear to differentiate between a cough and background noises, we need to actually define the characteristics of a cough and automate the identification of those traits using machine learning. We have started the process of looking at cough signatures from an open-access library online and begun to build our processing technique.”
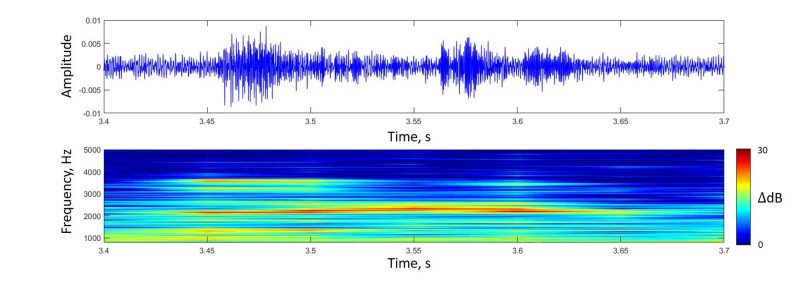
Recording and tracking coughs has previously been performed in hospital settings, but the processing of the acoustic data has traditionally been done manually. Through a multilayer acoustic identification approach using machine learning, the team will first identify auditory bursts, then search for the spectral content and time dependence of the signal learned from the library of coughs. Independent recognition of multiple acoustic traits will improve the identification process and reduce the number of false positives and false negatives.
The award funding will also support graduate research assistant Aaron Defreitas, who will work on the automatic cough detection software and coordinate the collection and analysis of cough samples. Defreitas, an aerospace engineering doctoral candidate, is studying anomaly detection methods in experimental data and plans to add an additional component based on this study to his doctoral thesis.
Once the software and processing algorithms have been developed and tested, the next phase will be to build a compact device to place in public spaces, such as a classroom or examination room. These devices, each composed of a microphone, computing engine, and data logger, will collect acoustic data and measure the number of coughs per hour based on the population of the room in an unobtrusive and privacy-sensitive manner.
Muelenaer, a practicing pulmonologist for three decades, has previously worked on audio signal classification in a clinical setting and will aid in the writing of an IRB for de-identifying data. Given that large clusters of new COVID cases are in institutions, connections with local long-term care facilities and the Virginia Department of Corrections medical staff will be leveraged to collect data in these locations. To ensure privacy, only non-speech audio snippets need be recorded for purposes of measuring cough frequency and classification.
“A coughing rate signal, which depends on geospatial location and time would be an important data stream for a statewide or national center for disease detection and forecasting,” said Ross. “It’s very important to bring together interdisciplinary teams with complementary expertise to tackle emerging problems like the current pandemic we find ourselves in.”
To support the Virginia Tech COVID-19 Rapid Response Seed Fund, visit the crowdfunding platform to learn more. Support will provide initial funding to faculty, staff, and students utilizing their expertise to investigate problems and help Hokie researchers advance the fight against COVID-19.
Written By Jama Green