New precision medicine approach could save patients grappling with life-threatening intestinal infections
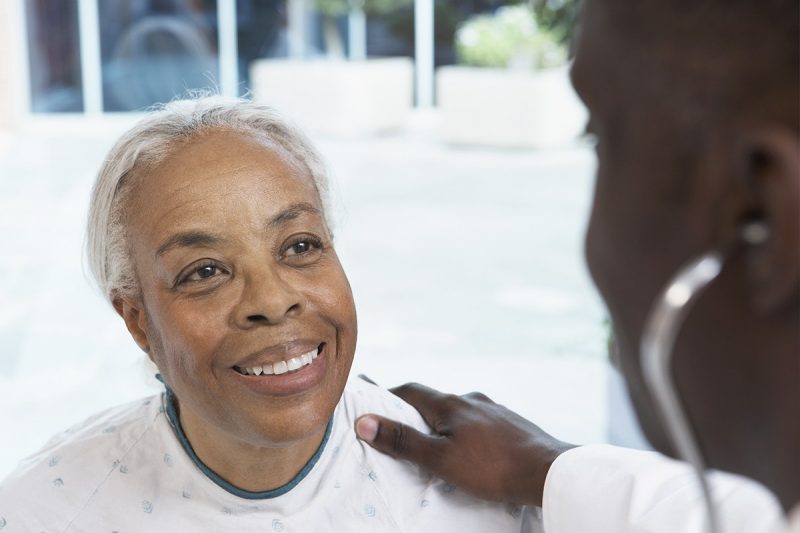
For years, patients in hospitals and nursing homes have faced an elevated risk of infection and even death due to a bacterium known as Clostridium difficile. In 2015, the Centers for Disease Control reported nearly half a million Americans had suffered from this disease — 29,000 of those cases resulted in death within a month of diagnosis.
To discover new treatments for this life-threatening intestinal condition, researchers at the Biocomplexity Institute of Virginia Tech have employed a combination of algorithms, simulations, and machine learning. These novel research techniques allowed scientists to make significant progress toward new precision medicine treatment options for patients suffering from C. difficile infections (CDI).
The Nutritional Immunology and Molecular Medicine Laboratory (NIMML), a leading lab at the Biocomplexity Institute, has developed a computational pipeline to test and predict the efficacy of existing and novel treatments for infectious and immune-mediated diseases. The pipeline incorporates mechanistic ordinary differential equation-based models with stochastic simulation and advanced machine-learning methods.
Researchers built upon an existing interaction model to develop the pipeline, which can now translate preclinical results in animal models to clinical outcomes, identify effective treatments, examine dosage effects, and predict patient reactions to combination therapies. The research study, recently published in Artificial Intelligence in Medicine, illustrates the development of a computational pipeline to test and predict the efficacy of existing and novel treatments for CDI.
“The convergence of advanced data analytics, modeling, and artificial intelligence systems with high resolution, large-scale patient data creates an opportunity to fundamentally transform how medicine will be practiced,” said Josep Bassaganya-Riera, director of NIMML and CEO of BioTherapeutics, Inc. “In this study and in our continuing efforts, we aim to be a leader in this developing field of precision, personalized medicine in infectious and autoimmune diseases.”
Modeling systems, such as this one, have the potential to revolutionize the design of treatment regimes away from the “one-size-fits-all” therapeutic approaches into a golden age of personalized disease treatment. Integration of patient characteristics from large-scale electronic health records can create virtual avatars to test new therapies and for predictive analytics to identify new molecular biomarkers capable of accurately predicting a patient’s response to treatment.
The ability to predict progression of disease and response to treatment is of particular importance in CDI. The majority of health care costs and mortality resulting from CDI are caused by high rates of recurrence that can exceed 50 percent of patients, depending on the treatment.
Antibiotics are currently the standard form of treatment for CDI, which run the risk of perpetuating drug-resistant bacterial strains, ultimately worsening the disease. Virginia Tech researchers say their new study points to a promising alternative treatment for CDI: a protein called lanthionine synthetase c-like or LANCL2.
“Our modeling shows that we do not need to remove the pathogen nor directly influence inflammation in the case of CDI to have an effective treatment,” said Andrew Leber, scientific director at BioTherapeutics. “Simply restoring immune tolerance in the gut through an LANCL2, or similar immunoregulatory pathway, or boosting the gut microbiome to allow it to naturally outcompete pathogenic C. difficile strains is effective in the absence of antibiotics.”
This study serves as a critical first step toward demonstrating the feasibility of constructing precision medicine tools that can optimize treatment design on a patient-by-patient basis. The application of these methods to personalized treatment of human diseases can potentially minimize undesirable side effects and maximize efficacy of treatment in response to C. difficile and other infectious and immune mediated diseases.
Editor’s note: This study is a part of a broader research collaboration between NIMML and the Translational Informatics Institute at Geisinger Health System, as participants in the national Precision Medicine Initiative Cohort Program.